If you have happened to see the mad rush that IT departments of large data-driven cultures are, you’d know that Business Intelligence requires a fast-moving infrastructure in place that can procure data, process it and throw decision-making insights. Self-Service Analytics is a collection of advanced technological and analytical platforms that empowers line-of-business professionals to implement Business Intelligence (BI) by performing queries and generating insightful reports on their own, with or without significant IT support. Self-service analytics comprises of easy-to-use BI tools with basic analytical abilities and underlying data models that has been customized and modularized for ease of understanding and elementary data access.
Why do we need Self-service analytics?
Internet of Things (IoT), Big Data Analytics and Cloud Technology are three related concepts that are transforming how manufacturing and industrial companies create value. These companies aim to change the nature of their relationship with customers by leveraging the strength of these technologies. Advancements in tech and design have facilitated the production of highly efficient devices at a lower cost. The number of devices connected to the communication networks is increasing and forming a complex and intricate network that can transmit and store a wide variety of huge information. This IoT network generates data that requires a new infrastructure to capture, process and store the same for further access. Cloud storage computing facilitates just that, while facilitating wider and easier access.
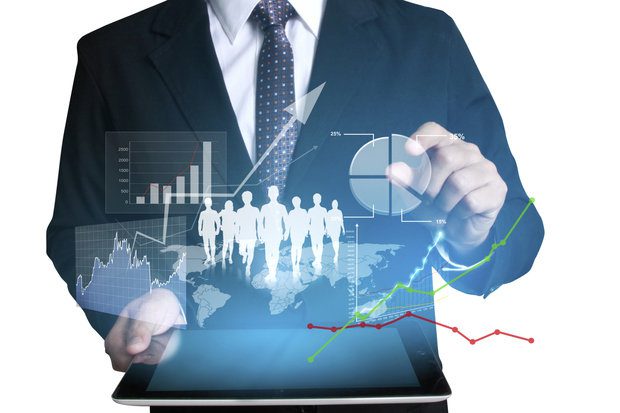
Furthermore, in order to use data for decision-making and value creation, it should be processed and analysed to extract insights. Data analytics and machine learning, backed by the expansion of computing power, enable companies to extract maximum value from data to build new offerings. The convergence of IoT , Big Data Analytics and Cloud Technology is creating a massive shift in the belief of value in individual objects to the dependence on interconnected devices and the information they generate. The shift from product orientation to information-based outcome orientation requires acquisition of certain new capabilities across a range of activities, from product development to sales. For instance, a company seeking to exploit opportunities in IoT and manufacturing smart devices, will require strong skillsets in data analysis and data management.
While a certain fraction of the current workforce might possess the skills to program devices and write advanced SQL queries to process data, many others would not. The need of the hour is hence, for a convenient-to-use and simple-to-understand platform that integrates the processes of procurement, processing, representation and storage of data. The conventional processes required people with a business acumen to design the solution, the implementation of the same was often done by an IT team adept at technological tools and processes. This not only slowed the process down, but also didn’t form a cohesive solution framework due to multiple stakeholders and their isolated understandings. Self-service analytics equips all individuals with the ability to design a solution and implement it without having to learn coding languages or Business-Intelligence specifically.
2017 is the year of growth of Self-Service Analytics
Analytics is a young industry still in the nascent stages of of growth. However, the evolution of the same has initiated adoption of higher impact-areas such as cloud-based Analytics-as-a-Service, Business Intelligence, the Internet of Things and Machine Learning. In recent years, cloud adoption has converted from a paper blueprint into the mainstream. It is now becoming the norm for many businesses and the benefits are clearly understood. The next upcoming buzzword in the cloud-technology space will hence be, Analytics-as-a-Service (AaaS). Running analytics on premise will be replaced by a comprehensive analytical solution is set to become a service at an operational cost.
As data analytics forms an integral core of the business framework, there will be a shift towards the business diving into data analytics combining software solutions. Databases like EXASOL, a data-preparation tool of the kinds of Alteryx, and a visualization tool like Tableau can be used to create a complete “Big Data analytics stack” can be created reducing time consumed and complexity in data analytics. This reduces the business users’ reliance on dedicated data scientists and IT resources. This allows them to run self-service business intelligence reports hence, cutting down on the resource-costs.
In 2017, the biggest developments in IoT seen to be stemming from the growing interest in industrial automation and smart cities and not just in smart devices. Thus, a more concerted effort will be observed in funding research into these two areas. Additionally, embedding machine learning and AI directly into the database will ensure that predictive analytics thrives with more advanced algorithms and drives better actionable insights. Prescriptive analytics is expected to increase its reach for providing the best recommendations to a business, based on data available. Ranging from retail to manufacturing and on to finance, usage of AI, along with the other above mentioned technological tools in business will be a big growth area.